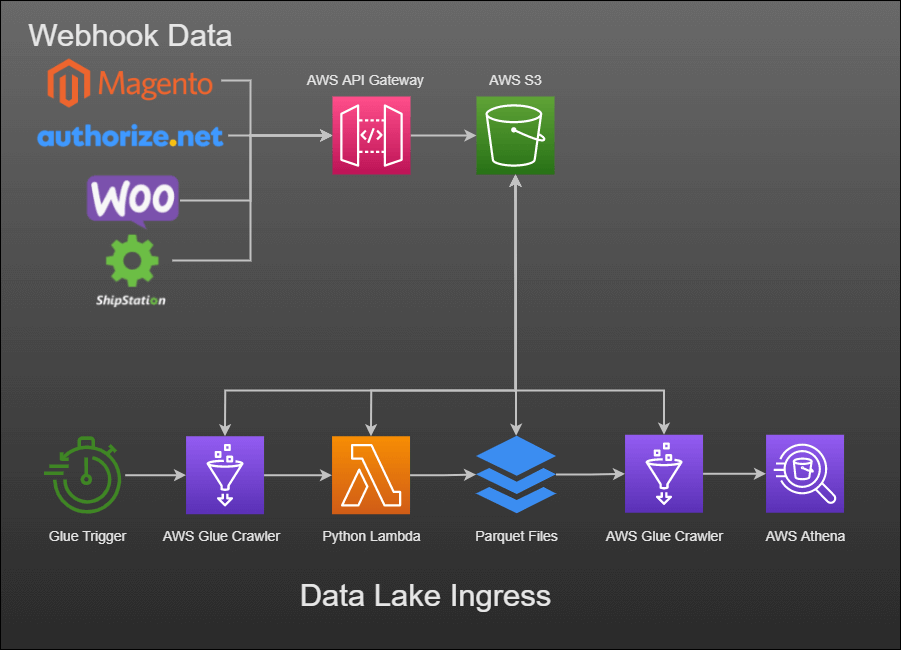
- application: Data Lake
- client: Product Manufacturer
- project date: March 2020 - April 2020
- summary: Data Lake ETL pipelines written in Python, leveraged AWS Glue, replaced a costly legacy process, and reduced AWS bill by 50%
Project Description
Background Info
JSON-encoded data was continuously collected through webhooks from various systems:
- eCommerce sites (WooCommerce and Magento)
- Payment gateways (Authorize.net)
- Shipping platforms (ShipStation)
This data then flowed through an ingress pipeline process and into a data lake.
Problem
An AWS cost analysis revealed high costs associated with that data ingress pipeline.
This pipeline consisted of:
- Parsing unstructured JSON-encoded data by resource-intensive jobs
- Translating into relational tables in PostgreSQL
- Retrieving the data back out of PostgreSQL
- Parsing the data into Parquet files for the data lake
Solution
The PostgreSQL AWS RDS instance and associated jobs needed to be decommissioned and replaced with AWS Glue ETL jobs better suited for translating irregular, unstructured data out of the data lake.
Contributions
In collaboration with my team, we established and refined the design and architecture of the new data pipelines.
I handled the shipping data while they handled the order and payment gateway data.
My shipping data pipeline did the following:
- AWS Glue Workflow: triggers a Glue crawler once a day
- Glue Crawler: crawls the S3 shipping data and groups it into tables and partitions
- Glue ETL: extracts those tables and partitions, transforms the data, and loads into Parquet files in another S3 bucket
- Glue Crawler: crawls the Parquet files from AWS S3 and loads into the AWS Glue Data Catalog
- AWS Athena: exposes the shipping data from the Data Catalog as Athena tables and views
The Glue ETL job was written in Python and ran in Apache Spark.
- Extract: Imported previously-crawled data
- Transform: Handed data conversions, resolved choices, and mapping
- Load: Wrote transformed data to Parquet files on AWS S3
Challenges Overcame
The biggest challenges were:
- Overcoming the learning curve for the internal movings parts: Glue crawlers, Parquet files, Data Catalog, Python libraries, and ETL jobs
- Figuring out the Python layer: which libraries to use, how choice resolution works, integrating with AWS S3 through Python, etc.
- Tracking down and fixing subtle data integrity issues using Jupyter Notebook
Accomplishments
- Helped establish new architecture and processes for data lake’s pipelines.
- Built my first production-ready Python code.
- Learned a lot about AWS Glue, ETL, data lakes, and Jupyter Notebook.
- Got to be a part of a 50% AWS bill reduction when the legacy RDS instance and pipelines were finally retired.
Technologies Used
- Languages: Python, JSON
- Concepts: Data Lakes, ETL Jobs, Data Crawlers, Glue Workflow, Webhooks
- Tools: pip3, PySpark, AWS Athena, Jupyter Notebooks
- Stack: Apache Spark, AWS S3, AWS Glue, AWS Athena